Written by Tommy Heck & Evan Claes
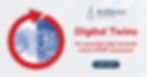
Digital twins driving smart factories
Industry 4.0 is currently revolutionizing the design, manufacturing and services of healthcare applications. It includes the automation and digitalization of manufacturing technologies, the acquisition and analysis of process data, and the communication between machines. Together, these innovations result into self-managed processes that turn traditional practices and manufacturing into so called smart factories.
An important aspect of these smart factories are digital twins. A digital twin is commonly described as a virtual replica of a physical product or process. It is a real-time computer model that can be used to predict process outcomes, optimize a process design, or monitor and control the production process. The digital twin receives real-time sensor data of the process and uses historical data or foundational assumptions (e.g., physical laws) to simulate the process evolution. In this way, both the efficiency and quality of the process can be increased, which also generates an important economic cost benefit.
Mechanistic and data-driven models
The computer models that are used in digital twins can be subdivided into so-called mechanistic and data-driven models. Both model types have their own strengths and weaknesses, and some digital twins may primarily or even exclusively use one or the other, depending on the application. However, the most successful digital twins typically use a combination of both. As will be discussed in the rest of this blog post, Antleron uses digital twins for the design and development of custom bioreactors for cell growth and for real-time, non-invasive monitoring of the cell growth during the process itself.
Firstly, mechanistic models are directly based on the underlying scientific laws of the process. For the example of cell culture in a bioreactor this means that culture dynamics are described by cell behavior (metabolism, proliferation and death) at the individual or coarse-grained cell level, while convection-diffusion equations are used to calculate the spatial distribution of nutrients in the bioreactor during culture. The biggest advantage of mechanistic models is that they can provide insight in the process because their parameters are based on the underlying physics. Therefore, a mechanistic model can also be easily adapted to, for example, a change in the bioreactor design. However, mechanistic models in general have a high cost of development, their parameters can be difficult to validate, and they can require large computing resources, which might hamper real-time computations.
The data-driven approach, on the other hand, uses collected process data together with machine learning models to build (part of) a digital twin. In contrast to a mechanistic model, a data-driven model does not rely on physical and biological knowledge of the system, but uses process data to build a mathematical model that can simulate the process. They are sometimes called ‘black-box’ models, as they provide little to no new insight into the underlying dynamics of the process, because the models only simulate patterns they learn from data but are not based on physical or biological principles. However, a major advantage of these models is that their development - provided that sufficient data is available - is fast and efficient. They can run in real-time with the process and their validation is relatively straightforward. The correlations they provide can for example be used to monitor the process critical quality attributes (CQAs) non-destructively and in real-time, an application which is also known as soft sensing.
Hybrid approach combines the best of both worlds
In between these two extremes of the modeling spectrum lies the hybrid approach that attempts to combine the best of both worlds. The fundamental idea behind this approach is to use the complementarity of the two opposing methodologies: a mechanistic process model can be used to model the part of the process about which established physical knowledge is available (e.g. fluid flow or nutrient distribution), while a data-based model is used to model the part of the process which cannot be modeled mechanistically because of the complexity or lack of available knowledge (e.g. cell growth kinetics). Such models offer an appealing trade-off between development speed and process insight. Additionally, they can outperform mechanistic and data-based models with regard to predictive power and applicability.
Digital twins play a central role in the digitalization and automation of manufacturing strategies, particularly for the field of advanced therapy medicinal products (ATMPs), which are medicinal products based on cell, gene and tissue engineering processes. When successful, digital twins can be used to fast-track process development by allowing scientists and engineers to run simulation experiments as part of the process development. These kinds of experiments can be carried out much faster and at a much lower cost than typical ‘wet lab’ experiments, for which process times and raw material costs can be exceptionally high.
Simulation-driven development is therefore a way for industry to lower manufacturing and process development costs. Once the process is deployed in manufacturing, digital twins can play a key role in process management by allowing to monitor the full process at every time point, and thus increasing the process robustness and manufacturing reliability through increased process control. For ATMPs, the process needs to be equipped with a flexible control strategy in order to ensure consistent product quality in face of significant raw material variability, in particular for autologous processes. On top of this, the industry is currently moving towards personalized manufacturing in which the end product is tailored to individual patient demands, further stressing the need for a high level of non-invasive process monitoring and control, which is unattainable without the power of a digital twin.
Digital solutions at Antleron
At Antleron, we work hard to develop next-generation bioprocess blueprints. In our research activities we use a set of digital tools (computational fluid dynamics, data-based and hybrid models) to allow process modeling and employ the data generated from experiments for optimization and soft sensor development. In combination with the quality by design methodology, this digital twin based approach reduces development timelines and allows for gaining deeper insights, ultimately leading to more robust and efficient cell-based bioprocesses.